Motion Analysis (WP5)
Motion analysis for musculoskeletal conditions have advanced significantly in recent years. This technology progressed to sophisticated systems that offer precise and detailed insights into human biomechanics. Traditional methods such as patient self-reports and practitioner observations have limitations in diagnosing and treating musculoskeletal conditions [1]. Advancements in computational technology have facilitated real-time estimation of ground reaction forces and joint moments using inertial measurement unit sensors, enhancing the understanding of gait and movement dynamics [2, 3]. These tools have significantly enhanced the objectivity and accuracy of musculoskeletal health assessments, leading to more effective clinical decision-making [4]. Musculoskeletal modeling has become a prevalent and valuable tool for studying and analyzing human motion [5], specifically for researchers to obtain valuable information about joint and muscle dynamics [6]. Current laboratory-based motion capture remains a key data acquisition technique for quantifying human biomechanics [7]. These models can estimate in vivo joint contact forces [8]. Motion analysis systems have traditionally relied on video analysis with sophisticated 3D motion capture technology in controlled laboratory environments, presenting several key limitations [9]. These limitations included limited accessibility due to confinement to specialized laboratories, high costs of equipment and the need for trained personnel, complexity requiring extensive training, and static environments limiting real-world applications [10].
The MAI Motion Technology® in comparison will transcend the mentioned limitations by integrating state-of-the-art holistic kinematic analysis with user-friendly, spreadable, real-world, reproducible and portable technology. It will enable the capture of full-body kinematics and dynamics data, enhancing the analysis of human motion in various settings This is achieved through the integration with mobile devices democratizing access to advanced motion analysis and making it feasible for use in diverse environments facilitating the collection of consistent and comparable data across different locations without the need for specialized infrastructure.
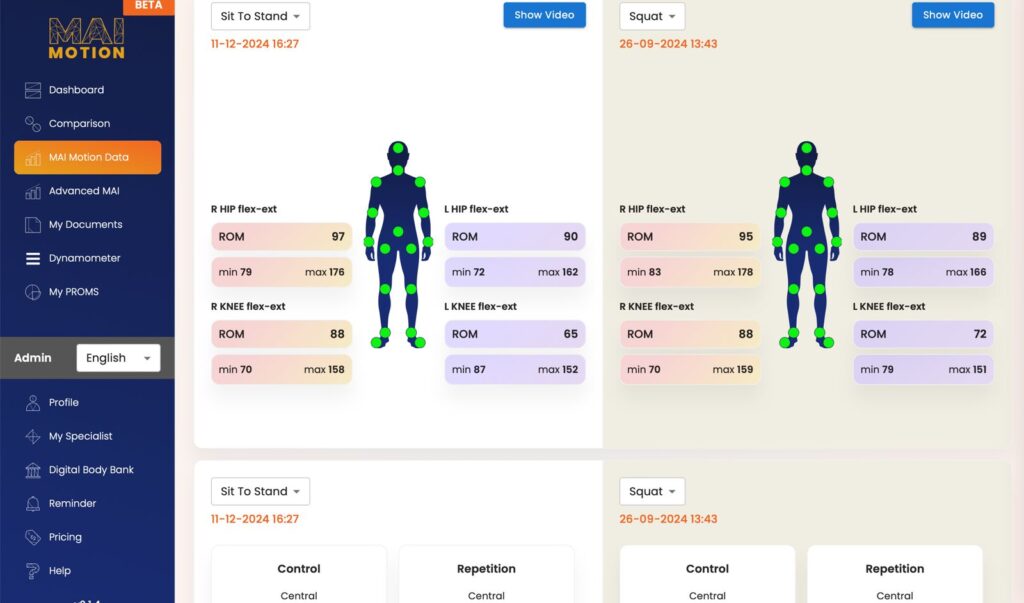
….
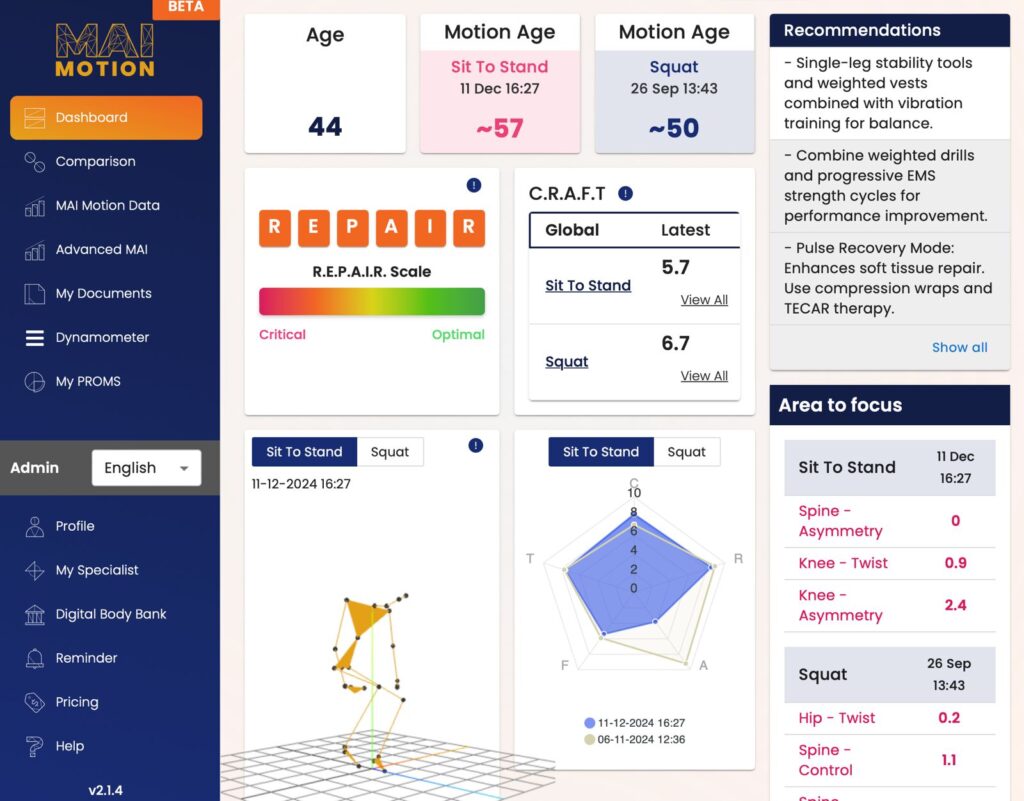
….
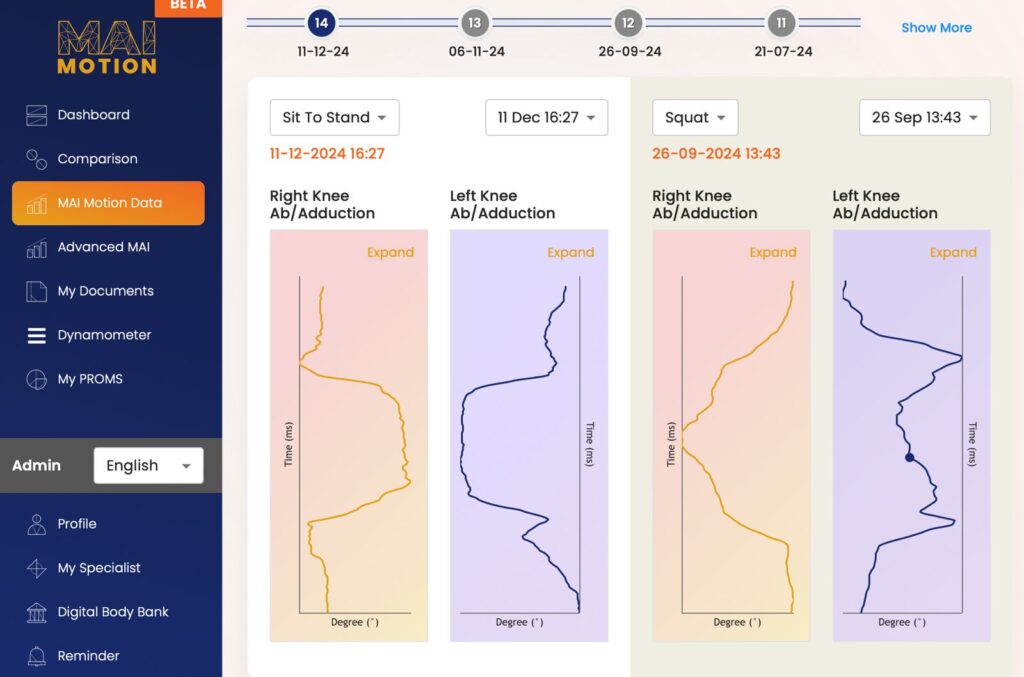
The MAI Motion Technology® transcends the limitations of traditional motion analysis systems by integrating state-of-the-art, holistic kinematic analysis with user-friendly, scalable, real-world, reproducible, and portable technology. It enables the precise capture of full-body kinematics and dynamics in various settings, enhancing the analysis of human motion without the need for specialised infrastructure such as dedicated motion labs or extensive sensor arrays.
At the core of this transformative system is the proprietary CRAFT (Control, Repetition, Asymmetry, Flow, Twist) framework, which offers an objective, data-driven approach to motion analysis. CRAFT provides structured evaluations of critical biomechanical parameters such as movement symmetry, joint stability, and efficiency, enabling clinicians and researchers to identify subtle changes in motion patterns. This capability is invaluable for monitoring progression or recovery following treatment or rehabilitation exercises.
The technology’s compatibility with widely available laptops, computers, and mobile devices democratises access to advanced motion analysis, making it feasible for use by healthcare professionals, researchers, and even end-users in diverse settings such as clinics, sports facilities, or at home. Its portability ensures the collection of consistent, high-quality data across environments while facilitating longitudinal monitoring and remote assessments.
The system’s unique ability to combine real-world usability with high-precision analysis ensures not only improved accessibility but also actionable insights. By enabling repeatable, non-invasive assessments, MAI Motion Technology® allows clinicians to objectively track patient progress over time, tailoring treatments to maximise outcomes and accelerate recovery. This capability is particularly advantageous for evaluating the effectiveness of interventions, providing a reliable method to measure improvements in biomechanics, movement efficiency, and overall function.
Reference:
[1] Wade, L., Needham, L., McGuigan, M., & Bilzon, J. (2022). Applications and limitations of current markerless motion capture methods for clinical gait biomechanics. Peerj, 10, e12995. https://doi.org/10.7717/peerj.12995
[2] Recinos, E., Abella, J. R., Riyaz, S., & Demircan, E. (2020). Real-time vertical ground reaction force estimation in a unified simulation framework using inertial measurement unit sensors. Robotics, 9(4), 88. https://doi.org/10.3390/robotics9040088;
[3] Hossain, M. S. B., Guo, Z., & Choi, H. (2023). Estimation of lower extremity joint moments and 3d ground reaction forces using imu sensors in multiple walking conditions: a deep learning approach. IEEE Journal of Biomedical and Health Informatics, 27(6), 2829-2840. https://doi.org/10.1109/jbhi.2023.3262164
[4] Halvorson, R. T., Castillo, F. T., Ahamed, F., Khattab, K., Scheffler, A., Matthew, R. P., … & Bailey, J. F. (2022). Point-of-care motion capture and biomechanical assessment improve clinical utility of dynamic balance testing for lower extremity osteoarthritis. PLOS Digital Health, 1(7), e0000068. https://doi.org/10.1371/journal.pdig.0000068
[5] Wibawa, A., Verdonschot, N., Halbertsma, J., Burgerhof, J., Diercks, R., & Verkerke, G. (2017). A trap motion in validating muscle activity prediction from musculoskeletal model using emg. International Journal of Bio-Science and Bio-Technology, 8(6), 61-72. https://doi.org/10.14257/ijbsbt.2016.8.6.07
[6] Chan, Y., Teo, Y., Gouwanda, D., Nurzaman, S., Gopalai, A., & Thannirmalai, S. (2022). Musculoskeletal modelling and simulation of oil palm fresh fruit bunch harvesting. Scientific Reports, 12(1). https://doi.org/10.1038/s41598-022-12088-6
[7] Werling, K. (2023). Addbiomechanics: automating model scaling, inverse kinematics, and inverse dynamics from human motion data through sequential optimization. Plos One, 18(11), e0295152. https://doi.org/10.1371/journal.pone.0295152
[8] Raimondo, G., Willems, M., Killen, B., Havashinezhadian, S., Turcot, K., Vanwanseele, B., … & Jonkers, I. (2023). Peak tibiofemoral contact forces estimated using imu-based approaches are not significantly different from motion capture-based estimations in patients with knee osteoarthritis. Sensors, 23(9), 4484. https://doi.org/10.3390/s23094484
[9] Latella C, Kuppuswamy N, Romano F, Traversaro S, Nori F. Whole-Body Human Inverse Dynamics with Distributed Micro-Accelerometers, Gyros and Force Sensing. Sensors. 2016; 16(5):727. https://doi.org/10.3390/s16050727
[10] McGinnis RS, Perkins NC. A Highly Miniaturized, Wireless Inertial Measurement Unit for Characterizing the Dynamics of Pitched Baseballs and Softballs. Sensors. 2012; 12(9):11933-11945. https://doi.org/10.3390/s120911933